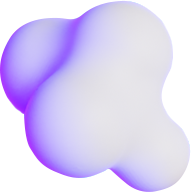
Kinsey Dardanus – Oct 1, 2020
Introduction
Bubble Sort is a worldwide leading puzzle-game, on both Android and iOS, totalizing more than 15 millions of players across the world. As a puzzle-game, deep players’ behavior analysis and level difficulty improvements were the keys to its success.
At TapNation, our data scientists and engineers have developed tools and algorithms to monitor, analyze and test our gameplay and difficulty curves in order to improve our KPIs. In this document, we will share some of the techniques and tips we used to make Bubble Sort a hit-game.
Boost Your Retention Using Data
When optimizing a game, you need to define your metrics. Are average retention and session-time the most important metrics? Are there any other ones?
Retention and session time are useful proxies for the main metrics when it comes to business: is the game profitable, and by how much? For Bubble Sort – a puzzle game – we concluded that CPI, session time and retention (relative and absolute) were not good enough to insure its profitability. We, therefore, included from the start ads to analyze their behaviors in a manner that would be similar to the one at scale. Therefore, we were directly able to optimize not only retention and session time, but Lifetime Value (LTV) itself!
By optimizing our Lifetime Value (LTV) directly, we managed two things: first, we found interesting new AB tests to optimize the user experience, and second, we saved a lot of time when launching the game at scale.
Actionable Data
In hyper-casual, don’t come up with preconstructed ideas! Players will always surprise you and that’s the whole fun of this business.
At TapNation we always think of how data can improve business, and how data can be used to shape new hypotheses on a game and how to test them. That’s why, for Bubble Sort, AB testing is entirely part of our creative process.
Streamlining our A/B testing process is one of the keys of TapNation’s success. For Bubble Sort, we launched more than 50 AB tests – and methodically analyzed their results. And the results were surprising, to say the least. Some small changes (UI and progression) increased our KPIs drastically, whereas some big gameplay changes did not add any value, and sometimes decreased our general Lifetime Value (LTV).
From Data Analysis to Data Science
Our Data Scientists used our craftily trained Lifetime Value (LTV) models to generate thousands of scenarios for Bubble Sort, in order to understand where we had to work the most to improve the game’s profitability. Our AI generated those scenarios, which were then analyzed by our product team and other members.
Those scenarios were generated in an iterative process, where at each round, our AI learned from the result of the previous one. For Bubble Sort, we have implemented those processes from the beginning, which both helped us increase retention and KPIs dramatically but also saved us time from useless gameplay changes. More precisely, those scenarios have helped us increase our Lifetime Value (LTV) by 18%.
Hyper-casual players come from different backgrounds. Especially for puzzle games where performances are very different across players and therefore the level curve must be custom shaped.
For Bubble Sort, we applied clustering techniques very early on in the development in order to find the best performing users for our Lifetime Value (LTV) and understand their progression paths. More specifically, we identified problematic levels and “churning patterns”. Our Data Scientists developed algorithms to analyze, visualize and share those patterns to the rest of our team members.
Conclusion
For Bubble Sort, we implemented a carefully crafted process which allowed us to get actionable data from the start, analyze and visualize those data points, share those results to all teams in order to quickly iterate and improve our game’s KPIs that led to a profitable HIT game.
Through simulations and clustering, our data-scientists and engineers have elevated the AB test paradigm one step further to design the finest hit game generator.